Probability distribution
This article is about the mathematics of Student's
t-distribution. For its uses in statistics, see
Student's t-test.
Student's t
Probability density function 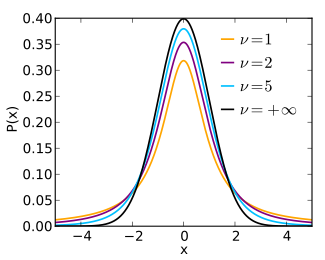 |
Cumulative distribution function  |
Parameters |
degrees of freedom (real, almost always a positive integer) |
---|
Support |
 |
---|
PDF |
 |
---|
CDF |
![{\displaystyle {\begin{matrix}\ {\frac {\ 1\ }{2}}+x\ \Gamma \left({\frac {\ \nu +1\ }{2}}\right)\times \\[0.5em]{\frac {\ {{}_{2}F_{1}}\!\left(\ {\frac {\ 1\ }{2}},\ {\frac {\ \nu +1\ }{2}};\ {\frac {3}{\ 2\ }};\ -{\frac {~x^{2}\ }{\nu }}\ \right)\ }{\ {\sqrt {\pi \nu }}\ \Gamma \left({\frac {\ \nu \ }{2}}\right)\ }}\ ,\end{matrix}}}](https://wikimedia.org/api/rest_v1/media/math/render/svg/32b4a3af11d075b054f564e60b7aea14bf2a95f3)
where is the hypergeometric function |
---|
Mean |
for otherwise undefined |
---|
Median |
 |
---|
Mode |
 |
---|
Variance |
for ∞ for  otherwise undefined |
---|
Skewness |
for otherwise undefined |
---|
Excess kurtosis |
for ∞ for  otherwise undefined |
---|
Entropy |
![{\displaystyle \ {\begin{matrix}{\frac {\ \nu +1\ }{2}}\left[\ \psi \left({\frac {\ \nu +1\ }{2}}\right)-\psi \left({\frac {\ \nu \ }{2}}\right)\ \right]\\[0.5em]+\ln \left[{\sqrt {\nu \ }}\ {\mathrm {B} }\left(\ {\frac {\ \nu \ }{2}},\ {\frac {\ 1\ }{2}}\ \right)\right]\ {\scriptstyle {\text{(nats)}}}\ \end{matrix}}}](https://wikimedia.org/api/rest_v1/media/math/render/svg/f248820e946d0f06d1d132fb0d1473d3bed1736c) where
is the digamma function,
is the beta function.
|
---|
MGF |
undefined |
---|
CF |
for
|
---|
Expected shortfall |
Where is the inverse standardized Student t CDF, and is the standardized Student t PDF.[2] |
---|
In probability theory and statistics, Student's t distribution (or simply the t distribution)
is
a continuous probability distribution that generalizes the standard normal distribution. Like the latter, it is symmetric around zero and bell-shaped.
However,
has heavier tails and the amount of probability mass in the tails is controlled by the parameter
For
the Student's t distribution
becomes the standard Cauchy distribution, which has very "fat" tails; whereas for
it becomes the standard normal distribution
which has very "thin" tails.
The Student's t distribution plays a role in a number of widely used statistical analyses, including Student's t test for assessing the statistical significance of the difference between two sample means, the construction of confidence intervals for the difference between two population means, and in linear regression analysis.
In the form of the location-scale t distribution
it generalizes the normal distribution and also arises in the Bayesian analysis of data from a normal family as a compound distribution when marginalizing over the variance parameter.